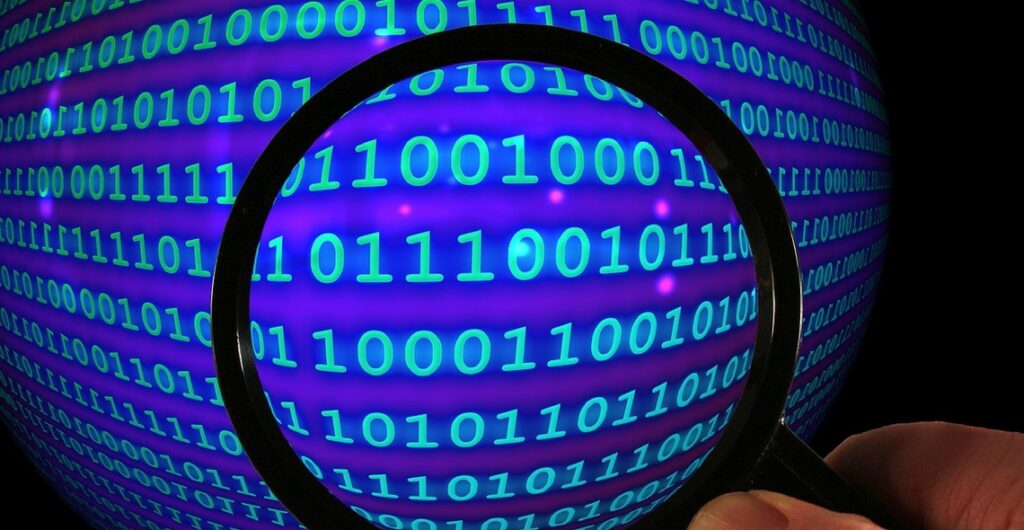
Posted 2nd December 2016
This is a blog post by Jeremy Nicholls, CEO at Social Value UK and Social Value International.
Whether data is rigorous enough is a common discussion amongst social impact analysts, social accountants and evaluators. And the great thing is that it is always possible to argue that it’s not. Which may be fun in arguments but becomes a massive problem if we ever want the group of people who we want to use this information to ever make a decision using it. And that’s all they hear, its not good enough, we need more resource to make it better, the sample size is too small, not representative, the approach to causality, assuming there is one for a moment, is inadequate and could even increase the risk and so on. The starting point needs to be the audience and an understanding of the decisions or decisions that may be informed by this information. So the audience could be a funder, the board, a management team, the general public and so on. The purpose could be to win a contract, get funding or for internal decisions. It could be to develop existing services or products, to target products at specific segments within a stakeholder group or to scale and to stop. What will be good enough will depend on:
- the audience and their understanding of the risks in the data; and
- the purpose
What is being assessed is the risk that the wrong decision is made. Those deciding will need to understand this risk. The quality of the data is only one of three factors that need to be considered. The three factors are:
- The quality of the data
- Consequences of deciding
- The time available for the decision
Data quality
There are many issues that effect data quality but there is often a focus on accuracy. The issues raised above on sample size, whether its representative and so on relate to data accuracy. Important but only one factor. There are three factors:
- Data accuracy
- Data completeness
- Level of aggregation
One of the biggest risks is that the data isn’t complete. Outcomes experienced by stakeholders whether positive or negative haven’t been included and so the decision maker won’t know what the effect of the decision on these non-included outcomes, potentially for example, increasing a negative outcome. Whilst is seems intuitive that negative outcomes (once it has been accepted these are being caused by the activity) should be included, it is often less obvious why other positive outcomes should be included. People want to design out any negatives, but designing for other positive outcomes (subject to any funding constraints) can increase value for stakeholders.
The next risk is that the decision will affect different people within a stakeholder group in different ways, depending on their characteristics. At one extreme, this would mean having data on each individual but some level of aggregation will help decision making and be a reasonable grouping of shared outcomes and characteristics. If impact data is generally being collected for funders and consequently very aggregated, there is a risk that it won’t have the level of detail necessary for internal decision making. There is also a worry that data collection systems are not designed to allow for enough, if any, segmentation.
Recognising these risks raises the question of how decision makers get assurance that the data is good enough. Firstly, through a discussion between those making decisions and those designing systems and collecting data, so that decision makers have confidence in those systems. Secondly, through checks after the data has been collected that accuracy, completeness and segmentation have been considered.
Consequence of deciding
The consequence of getting it wrong don’t seem to be considered. The discussion stops once someone argues the risk is too high (and risk of accuracy more than anything else). But it shouldn’t stop yet. The next issue is to think about the consequences and again there are three factors:
- The resources that will not be lost
- The cost of reversing the decision to the organisation
- The costs incurred by other stakeholders that have been effected
Clearly for major capital expenditure programmes the risk needs to be lower. For a small development of a product, which can perhaps be piloted, the resources may be much lower and so the level of acceptable risk higher. Similarly, the cost of reversing a major capital project, or redeveloping some sections will be high. The cost of reversing a pilot enhancement or different way of delivering would be zero. Again a higher level of risk can be accepted. And as well as costs to the organisation, it is possible that others will be effected. A change to an employment programme that may be easy to reverse but resulted in fewer people gaining work could represent a high loss of value to some people.
Available time
Finally, there is the practical issue of the amount of time available. A programme evaluation where the aim may be to influence next year’s programme choices has more time than a decision which needs to be made in the next couple of weeks before external factors make the decision redundant. The shorter the timescale for the decision the lower the quality of the data and the higher the risk.
Why make a decision (based on this data)?
Even when the data is good enough and the decision makers agree, that doesn’t mean that a decision will be made. The other factor is to consider the pressure to decide. Leaving things as they are is easier than making changes. A culture of testing, trial and error, and rapid prototyping will make things a lot easier. In the end, you might just measure the number of proposals to improve, stop or scale services, the number tested and the number still running.